The Sedona Conference WG13 on AI & Law: Part 1
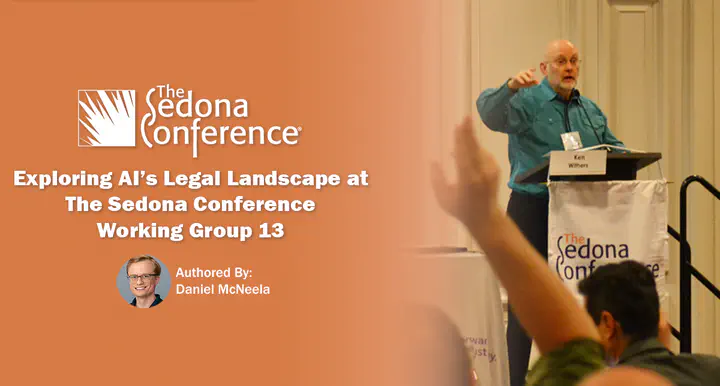
Introduction
Last week, I had the unique opportunity to attend the inaugural meeting of The Sedona Conference Working Group 13 on Artificial Intelligence and the Law. For those who are unfamiliar with it, The Sedona Conference (Sedona) is a 501(c)(3) research and educational nonprofit dedicated to advancing the law in new and burgeoning areas. The vehicles by which Sedona accomplishes this are its working groups, which bring together attorneys, judges, scientists, engineers, academics, and policymakers for open discussion and dialogue on topics as varied as patent litigation best practices (WG10), data security and privacy liability (WG11), trade secrets (WG12), and, most recently, AI and the law (WG13). While Sedona certainly has a current bent towards issues in intellectual property, past working groups have covered topics as wide-ranging as discovery, mass torts, and antitrust.
The thrust of the Sedona Working Group model is this: after convening, legal experts engage in open and unfettered dialogue, bringing to bear their diversity of experiences and professional needs relating to emerging legal issues. They do this in order to suggest updates and revisions to existing law as well as provide guidance and best practices for the judiciary and attorneys within a given subfield. Panels of experts are also invited by the Working Group’s Steering Committee to moderate the discussion surrounding particular subtopics, but input is welcomed from everyone in attendance, and all opinions are valued equally. After the general session of a working group concludes, those who would like to further their involvement in Sedona’s activities are assembled into a series of democratic committees, carefully balanced to ensure all viewpoints are represented, which reconvene throughout the year to codify concrete recommendations. Once finalized, these recommendations are published by The Sedona Conference and distributed widely.
Luckily for me, I attended the inaugural meeting of Working Group 13, which gave me the opportunity to observe the unique groundswell of excitement building around the impact of AI, in particular generative AI, on the legal field. While previous Sedona working groups had tangentially touched on AI’s impact, they had done so under the umbrella of other topics such as data security and privacy liability. However, the recent popularization of generative AI tools such as ChatGPT convinced Sedona that a dedicated working group on AI was necessary.
The conference kicked off with a welcome reception at the Camby Hotel in the Biltmore Area of Phoenix. The first thing I noticed upon arriving was the camaraderie of conference attendees. It quickly became apparent to me that many participants had become friends as a result of their participation in past working groups, from having worked together in law firms, e-discovery and legal tech startups, and even from appearing as opposing counsel in court. Seeing this impressed upon me the collegiality of the legal profession and counteracted the oft-parroted idea that law is an adversarial practice; while the American judicial system is inherently adversarial, that does not mean that the oppositional nature of the law extends outside of the courtroom. I was also heartened to discern a distinct lack of hierarchy – it was not uncommon to see associates chatting with district court judges and attorneys socializing with serial entrepreneurs. For my own part, I was fortunate to talk with a number of attorneys in the IP space and learn about their personal experiences in the field of patent law, specifically patent litigation, in which I aim to practice. I appreciated the chance to get advice from successful practitioners, and I left the welcome reception feeling reaffirmed in my decision to pursue intellectual property law.
The next day marked the start of the official conference proceedings and kicked off with an introduction by Kenneth Withers, the Executive Director of The Sedona Conference. Ken emphasized the influence of Sedona, mentioning how the recommendations of its working groups have been cited in numerous judicial opinions, including those of the Supreme Court. He also introduced us to the famous Chatham House Rule, which has been adopted by Sedona in order to foster an open dialogue among conference participants. Basically, the rule posits that conference attendees can use the information discussed in any way they see fit except that they cannot attribute any quote to any particular individual; the identities of speakers are not to be tied to any statement, and privacy is to be strictly preserved. Ken mentioned that the conference has never had to address even a single violation of this rule, suggesting that it has been well-received and implemented by attendees for many years. To that end, while I will identify panelists (these are publicly available on Sedona’s website) in this post and speak in generality of what was discussed, I will not divulge who held any specific opinion, and I will not directly quote anyone.
What Do We Mean by AI?
The discussion commenced with a panel that was aptly titled “What Do We Mean by AI?” The panelists guiding the conversation included Paul H. McVoy, the SVP of Repario; Niloy Ray, a shareholder and litigator at Littler Mendelson; Dr. Harry Strange, a PhD in machine learning and a Senior Associate at Withers and Rogers; and Lucky Vidmar, Associate General Counsel at Microsoft. The purpose of this panel was, broadly speaking, to get everyone on the same page regarding the terminology and technicalities of AI. Because so many different backgrounds and perspectives were represented in the room, it was important to lay a common framework of knowledge about AI that attendees could look to when subsequently dialoguing about more intricate issues.
The necessity of this panel quickly became apparent. As people began asking questions and engaging in discussion, it became clear that attorneys, engineers, and scientists frequently used the same words to describe distinct phenomena. For example, while attorneys and judges were inclined to understand “model bias” as indicating the tendency of certain AI models to produce predictions that favored one demographic over another, engineers and computer scientists more readily viewed bias in the strictly statistical sense, that of the bias of an estimator (see e.g. the bias-variance tradeoff), i.e. how the expected value of a model’s predictions of a parameter can often deviate from that parameter’s true value.
The panel helped to bridge these gaps in understanding, as well as provide a general history of the development of AI. For example, the panelists discussed how what we call AI has changed over time, from the human-driven symbolic logic and expert systems approaches of the early days (what they dubbed “old-fashioned AI”) to the data-driven AI approaches of today, exemplified by machine learning and deep learning.
Next, the panel discussed how the law is beginning to change to meet new challenges introduced by the latest wave of generative AI and large language models (LLMs). The general consensus was that much of the emerging law centers around addressing algorithmic, or model, bias, in the sense of a model promoting disproportionately favorable or unfavorable outcomes for a given demographic. One point the panelists stressed, and which I agree with, is that there is really no such thing as algorithmic bias, as models are simply trained using the neutral principles of mathematics and statistics to reproduce probability distributions. In reality, models can become biased when they are trained on biased data, such as an imbalanced training set that insufficiently represents a subset of a population. While there is no perfectly unbiased dataset, larger datasets tend to exhibit less bias as they are more representative of the general population.
Biased models can obviously lead to poor and unwanted legal outcomes. For example, models that assess creditworthiness can run afoul of financial regulations or laws prohibiting discrimination on the basis of race, sex, and religion. Similarly, as AI models become a more integral part of the hiring process, there is increased risk that a biased model could violate extant employment law. One particularly trenchant concern was that models might soon be used in making criminal sentencing decisions, and that this could potentially lead to prejudice against, and deprivations of due process for, minorities who have historically faced discrimination. However, others expressed optimism that such models could help to provide a fairer shake to criminal defendants based on statistically significant outcomes, such as a person’s predicted chance of reoffending, and help to eliminate the silent biases of individual judges.
There was also spirited debate as to whether judges and attorneys should approach AI models with the presumption that they’re biased or, rather, perform an ex-ante analysis of bias based on whatever differential outcomes might result. Given the fact that bias can’t be completely eliminated, some advocated for guidelines that could be used to determine whether a model exhibits an “acceptable” level of bias. Others voiced a somewhat antipodal concern – if humans are inherently biased, and models are designed to pick up on that bias as it manifests in data, should policymakers and the judiciary proactively be seeking to correct that bias? At what point does a judge cross the line from ensuring the equitable treatment of participants in the legal system to putting their thumbs on the scale and imposing a personal set of normative judgments that have not been adopted by the society at large? Would the correction of model bias, absent some legislative policy to the affirmative, overstep the bounds of the judiciary so as to be unconstitutional? These are all questions that WG13 will have to grapple with as it seeks to craft more concrete proposals and recommendations.
Next, the discussion shifted towards the regulation of AI and laws seeking to impose such regulation. Many noted that the push for regulation seems to stem from a certain fear factor that’s resulted from widespread public speculation about the potential emergence of AGI somewhere down the road. The panelists gave a broad overview of current AI regulatory frameworks. They mentioned that in 2019, California passed SB 1001 which mandated the disclosure of the use of chatbots for online platforms based in the state. In contrast, India and China have not yet passed any concrete laws, but are working to craft general-purpose legal frameworks around AI. It was noted that one of the challenges facing governments generally is the difficulty of even defining what AI is. For example, the UK government attempted to define AI for the purposes of its legislation and eventually settled on the somewhat vague answer of “products and services that are ‘autonomous’ and ‘adaptable’,” though many feel that this provides a less-than-satisfactory characterization. Meanwhile, a Biden administration executive order from 2023 defines AI as “a machine based system that can, for a defined set of human-defined objectives, make predictions, recognitions, or decisions.” Likewise, in 2021, the European Union passed the EU AI Act, the first significant regulatory framework surrounding AI; yet, it remains to be seen how such legislation will impact the use and development of AI technology, as businesses are still grappling with how to comply with multiple, often differing, sets of regulation. The first panel concluded with general debate around the scope of, and the policy decisions underlying, AI regulation.
Regulation of AI
The discussion concluding panel one led nicely into the start of panel two on the regulation of AI. This panel featured as speakers Brent Arnold, a partner at Gowling WLG Canada LLP; Karen Eltis, Professor of Law at the University of Ottawa; Micaela McMurrough, co-chair of Covington & Burling’s Technology Group and AI & IoT initiative; Niloy Ray, of Littler Mendelson; and Nichole Sterling, partner at BakerHostetler. The conversation was led by Dean Pelletier, founder of Pelletier Law in Chicago. The session picked up right where the first panel left off, and the panelists dove into discussion of the differences in regulations at the local vs. state vs. national levels. The general consensus was that implementing regulations at the local level would be unwise and would likely create erratic divergences in policies across jurisdictions, thereby disincentivizing the use of AI. Furthermore, there was general agreement that transnational and global businesses would have a difficult time mitigating their potential liability for the use of AI if too much regulation is imposed at the state level. Participants in the discussion emphasized that the purpose of the law is to serve the public by creating guidelines that are consistent, reliable, and predictable in their assessments of liability; too much or too fine-grained regulation could subvert this ultimate goal.
From there, the conversation delved into the nuances of assessing and allocating liability – should liability fall on the developer of an AI system, on the business that deploys the AI in their products and services, or on some combination of the two? Myriad approaches have been tried, each with their own set of advantages and disadvantages. For example, the EU AI Act apparently accords different responsibilities to the developer and the deployer of an AI tool, whereas current California regulations impose joint and several liability on the two parties.
Discussion next turned to the NIST AI Regulation Framework. Fundamentally, this framework posits that risk management should be incorporated by design into AI processes. This parallels the approach of risk management frameworks on the cybersecurity side and further introduces a reasonableness standard for risk management and governance.
A commonality among most existing regulatory frameworks is a delineation of AI use cases into categories of low, medium, and high risk. Most such regulations focus primarily on the high-risk use cases, particularly those which pose a threat to life and limb as in autonomous vehicles, medical diagnosis, certain types of robotics, and military applications. High-risk use cases are unique in that the level of tolerance for model bias and mistakes is low-to-nonexistent, and these provide the most fertile ground for strict regulation.
Panel participants also discussed regulations and recommendations within the privacy regime. For example, it is now commonplace for organizations that train LLMs and other generative AI models to allow users to opt-out from having their personal data incorporated into a model’s training set. However, this approach introduces thorny practical and ethical issues of its own. For example, if a singular demographic ultimately opts-out from model training en masse, could this then imbue the model with an impermissible bias against that same demographic?
Another point receiving frequent mention was that of the black-box nature of the neural network models powering modern AI. While the opacity of these models is permissible in certain applications, it poses unique challenges for legal use. While these models make highly accurate predictions, it is not usually possible to interrogate the model in order to understand the factors or logic that caused it to arrive at a given decision. This is particularly problematic in areas such as criminal sentencing, for obvious reasons, and in employment law, where the criteria used to arrive at an employment decision are often, legally-speaking, far more important than the ultimate hiring decision itself.
Under some existing regulatory acts, a person has the right to demand an explanation of how a decision about them was made and what they could have done differently to have secured an alternate decision. However, such requirements are pointless if the AI models being used are insufficiently explainable. Thus, further technical progress is needed to build truly explainable AI models whose decision making processes can be transparently audited for fairness and reproducibility. Anything short of this could amount to a deprivation of due process of law.
AI in Practice: Real-World Applications
The next panel pivoted from the technicalities of AI regulation to the discussion of creative uses of AI within the legal field. The panelists were Scott Bien, Vice President of Legal Solutions at Integreon; Luc Dahlin, Associate General Counsel in the IP Legal group at Meta; Tara Emory, Special Counsel at Covington & Burling LLP; and Jerry Ma, Director of Emerging Technology and Chief AI Officer at the U.S. Patent and Trademark Office (USPTO).
The panelists were quick to point out that individuals are now democratizing the power of AI in a way that wasn’t possible prior to the commoditization of generative AI products. End-users are utilizing AI to expand their creativity, to streamline previously inefficient workflows and processes, and to magnify their productivity and impact.
The conversation initially focused on the divide between individual (clients, attorneys, judges, etc.) and enterprise (law firms, e-discovery teams, etc.) use cases of AI. Human interaction (e.g. using AI as an assistant) is a major strength of current AI models, allowing, among other things, judges to efficiently schedule their dockets and outline opinions and attorneys to quickly draft memorandums and responses. Many conference participants contributed their own, unique AI use cases and expressed enthusiasm for its potential to transform the practice of law and eliminate much of the low-level drudgery that was previously the bane of practice. That said, its use was qualified by the need for caution and review, especially for judges and attorneys in court, as the potential for AI models to hallucinate was lost on no one, particularly given the notoriety of instances in which attorneys were sanctioned for presenting to the court briefs that contained hallucinated citations to nonexistent case law.
Discussion then shifted to enterprise applications of AI. Much of the enterprise focus seems to be on facilitating the ability for employees to interface with the large, internal knowledge bases of monolithic organizations. Of course, one particular appeal of generative AI for law firms and litigants is its ability to rapidly consume and summarize large swaths of text data. Thus, these AI tools are seeing increasing application to e-discovery and large-scale document review. Conference participants expressed excitement at the utility of generative AI for finding references to existing case law, for large-scale document search and summarization in discovery, for summarization of deposition transcripts, and for certain commercial contracting use cases, such as extraction of particular contract details and subsequent storage in a structured data format.
One recurring concern centered on determining how the legal billing model will need to change in response to AI, as some hypothesized that increased use of AI might signal the death knell of the billable hour. Yet, others predicted more modest, but still significant, changes to legal billing practices. Another major concern revolved around how AI might affect the education of young attorneys. While some worried that AI might displace or eliminate a substantial portion of the entry-level work that was crucial for educating the previous generation of lawyers, others expressed optimism that AI might present opportunities for new attorneys to take on more substantive and fulfilling work early in their careers.
To Be Continued…
In the next post, I will cover The Sedona Conference discussion around topics such as the monitoring of AI tools, AI governance, and the intersection of AI and copyright law. Stay tuned!