The Sedona Conference WG13 on AI & Law: Part 2
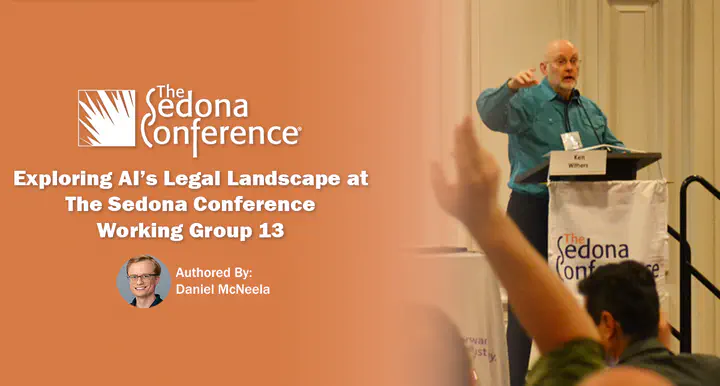
In my previous blog post, I covered the first three panels of the inaugural Sedona WG13. If you haven’t read it yet, you can do so here!
Measurement, Defensibility, and Compliance: Vetting, Validation, and Monitoring of AI Tools and Uses
The afternoon of WG13’s first day kicked off with a panel discussing validation and monitoring of AI tools. This panel featured Professor Gordon V. Cormack of the University of Waterloo, Dr. Eric Dunleavy of DCI Consulting Group, Professor Maura Grossman, also of the University of Waterloo, and Bruce Hedin of Hedin B Consulting.
A recurring theme of this panel’s discussion was the recognition of the need for robust industry benchmarks that could be used to evaluate AI models on relevant tasks. It is well-known that though LLMs are typically evaluated on a suite of academic benchmarks, performance on these doesn’t always translate to performance on the sorts of real-world tasks that practitioners care about. Especially in a field as fact-sensitive as the law, it is becoming increasingly important that lawyers, judges, and those with expert legal knowledge contribute to establishing gold-standard benchmarks that can be used to assess the performance of emerging AI models. The panelists and audience members identified some key deficiencies of current evaluations that should be addressed going forward: reliability (i.e. measuring a model’s consistency over time), validity (whether a model’s output is ‘correct’, in the legal sense) and fairness (whether the model unacceptably favors one demographic over another).
The panelists also echoed sentiments from the day’s previous panels, namely that no model will ever be perfect. Thus, it’s important for practitioners to establish acceptable thresholds for risk and precision in a given circumstance. The panelists also discussed whether the onus for critically evaluating model performance should fall on model providers, intermediate vendors, or the end users of the product. While the future will likely see evaluation duties distributed across all three parties, the panel highlighted that in order to stave off potential litigation arising from model failures in real-world settings, increased responsibilities will likely fall on the model providers and third-party vendors involved.
AI Governance
The next panel centered on the topic of AI governance. Admittedly, this is the panel I have the fewest notes on and which was most out of my wheelhouse. That said, the panel noted that many large companies such as Google are developing in-house corporate governance departments focused on addressing emerging issues around accountability and risk in the face of technological developments. This trend of increasing focus on corporate governance issues is expected to continue in the near future as AI technology becomes further integrated into business systems. There was also discussion about what constitutes an effective “governance stack,” namely, a corporate charter, a committee, an IP policy, and a use case approval process. Such a stack allows companies to put in place the administrative tools and processes necessary to reduce the risk of corporate liability down the line. Some conference participants characterized this under a duty of care risk analysis, holding that corporations perhaps owe a duty of care to their customers, shareholders, and employees and thus need to put safeguards in place to protect the respective interests of these parties in the face of technological risks.
Navigating the Litigation Landscape: Generative AI and Copyright Law
The panel on generative AI and copyright law generated palpable excitement among conference-goers, as this has been perhaps the most controversial and publicized of all issues at the intersection of AI and the law. Excitingly, the panel featured two panelists working at the heart of the controversy between tech companies and copyright holders: Holden Benon, an associate at Joseph Saveri Law Firm, LLP who is representing book authors in an ongoing class action litigation against OpenAI and Meta based on defendants’ training of large language models on plaintiffs’ copyrighted work, and Joseph Gratz, a partner at Morrison Foerster, who currently represents OpenAI and Stability AI in all of their pending U.S. copyright litigation. Panelist Mark Selwyn, a partner at WilmerHale and a co-chair of their Intellectual Property Litigation Practice Group, guided the debate while providing neutral analysis. Finally, Nikki Vo, a Director and Associate General Counsel at Meta Platforms, acted as the panel’s moderator.
The panel began with an overview of copyright law and, in particular, a refresher on the elements of a fair use defense to claims of copyright infringement. Fair use is assessed on a case-by-case basis. When assessing a fair use defense, the Court looks to four factors:
- The Purpose and Character of the Use
- The Nature of the Copyrighted Work
- The Amount or Substantiality of the Portion Used; and
- The Effect of the Use on the Potential Market for or Value of the Work
The panelists presented many of the arguments on both sides in the ongoing litigation around generative AI and copyright, particularly with respect to each of the four fair use factors. Under the first factor, current copyright law allows the use of copyrighted materials when that use is “transformative,” i.e. when it adds something new to the purpose and character of the original work. Fair use defenses are often successful in cases where the copyrighted material is used for such purposes as academic criticism, education, and parody. On the defendant’s side, the panelists analogized to the famous Authors Guild, Inc. v. Google, Inc. case, in which the Court found that Google’s digitization and indexing of the world’s books in their Google Books platform constituted fair use. On the plaintiff’s side, the panelists pointed out that there are a number of differences between training a large language model on the entire corpus of human authorship and what Google did in the Authors Guild case. Google’s use incorporated texts only for the purpose of enhancing Google search, but the text of copyrighted material remained invisible to end users outside of selected “preview” snippets from each work. In contrast, large language models and other generative AI tools can sometimes unpredictably regurgitate portions of their training data. Furthermore, a large language model can ingest the entirety of human written expression in a matter of hours. It can be argued that this goes far beyond the metes and bounds set by the fair use doctrine, which was established with the limitations of a human readership in mind.
The second fair use factor looks to the nature of the work, i.e. whether it is fictional or factual. Generally, the more creative a work is, the greater the copyright protection afforded it. Thus, works of fiction tend to receive greater deference than those of non-fiction under current copyright law. To establish the creativity underlying a copyrighted work, the plaintiff may present evidence of the skill that he has brought to the creation of the original. The Court will also look to whether the author has had the opportunity to enjoy the exclusive control and use of his work. In this case, the plaintiffs may argue that because they hold copyrights on works of fiction, the presumption should be against fair use. In opposition, the defendants might argue that the training process of a large language model simply extracts factual information from the creative works, and the underlying facts are not protected by copyright. The LLM then simply assembles these facts into its own creative expression in response to user prompting.
The third factor looks to the amount and substantiality of the defendant’s taking. Specifically, the Court might look at how much of a book (as a percentage of the total text) is being used to train the AI model. Because, as far as I’m aware, the entire text of any given book is fed as training data to an LLM in most training procedures, this factor is likely to weigh against the defendants. Another issue the Court is likely to analyze under this factor is the provenance of the training data, i.e. how it was obtained, and whether the party asserting fair use is acting in good faith. It has been alleged that many companies training LLMs obtained their training data from unlicensed channels, which could cast doubt on claims of good faith.
Finally, under the fourth factor, the Court will look to the effect of the use on the potential market for or value of the work. In doing so, the Court will assess whether there is evidence of actual or likely harm to the plaintiffs arising out of the unauthorized use of their work. Many past decisions have considered this to be the single most significant factor in the fair use analysis. The key consideration is whether it is likely that the derivative works could serve as a market substitute for the original works, i.e. could the outputs of large language models substitute for the books upon which those models were trained? For non-fiction works, this certainly seems possible, as products such as OpenAI’s Deep Research are already being used to generate full-length academic papers and research reports on every topic imaginable. For fiction works, the future is less certain. At present, large language models are typically less creative than humans and have not (to my knowledge) been used to generate full-scale works of fiction. However, this is likely to change in the future as model capabilities rapidly improve, and it is conceivable that LLMs will serve to contract the market for human-authored works of fiction in the coming years.
After discussing the fair use factors, the panel’s discussion turned to possible remedies in copyright battles of the kind currently being waged in the courts. Courts could potentially award monetary damages for past harms, injunctions against future training on certain types of data, or licensing arrangements that pay out to authors each time their copyrighted works are used to train an LLM. Settlement agreements might also lead to arrangements in which parties are allowed to opt-in to or opt-out from having their copyrighted works used in model training.
Until Next Time…
Stay tuned for the final post, covering AI as it applies to legal issues such as patenting, the judiciary, and future impacts, coming soon!